Incorrect data is expensive. And also problematic. Find out what data quality is and how good your data needs to be.
Who has not heard of it?
Name: Am Mustergraben 8
Telephone number: 000-0000-000
Date of purchase: 32/32/32
...
The list of incorrect data is lengthy and the problems and costs of poor data quality are an everyday reality in the German corporate landscape: from not reaching customers to the wrong address in a newsletter to incorrect invoicing - to name just a few examples. Following the "garbage in, garbage out" principle, bad data leads to bad decisions. Experian Marketing Services found out the following: 73% of German companies think that inaccurate data prevents them from offering an outstanding customer experience. Ensuring good data quality is therefore crucial for a company's day-to-day activities and, above all, a key success factor - and not just for data science projects. But what does data quality actually mean, how good does data have to be for a data science project, what are data quality criteria and can companies measure data quality? We will answer these questions in this article.
What is data quality and why is data quality so important?
Data quality Definition: In general, data quality refers to how accurate, complete, consistent and up-to-date data is. High data quality means that the data is free of errors, inconsistencies and outdated information. Low data quality leads to incorrect findings and poor decisions based on inaccurate or incomplete data.
However, data quality also describes how well data sets are suitable for intended applications. In this context, we therefore also speak of "fitness for use" - i.e. the suitability of the data for its intended purpose. This ensures that the quality of data is very context-dependent. Whilst the data quality may be sufficient for a certain application, it may be insufficient for another.
And why is it so important? Data quality is the basis for companies to have trustworthy data for analyses, business processes and decision-making. In a data science project, everything is based on data as a resource. In the project, data from various sources is brought together and then analysed. This data therefore serves as input for any analysis model. A sophisticated algorithm is therefore useless if the quality of the data is poor. Even if a data science project can fail for a variety of reasons, the success of the project depends primarily on the quality of the available data. Please also read our article "How to master data science projects".
You are well advised to invest in measures that ensure the high quality of your data. On the one hand, this is crucial for the success of the project, but also beyond that. After all, a lack of data quality can result in considerable (consequential) costs for a company. Let's take a look at this.
Poor data quality has multiple costs
Poor data quality has a name: Dirty data. This data is characterised by low values when it comes to consistency, completeness, accuracy and timeliness. Here are some facts about the monetary impact of these circumstances:
- Gartner estimates that the average company loses up to 15 million US dollars in revenue due to faulty data (Gartner's Data Quality Market Study). In other words, the cost of poor data quality is 15% to 25% of your revenue (study published in MIT Sloan Management Review).
- 50% of the IT budget is spent on reprocessing data (Zoominfo).
- Once a data series has been recorded, it costs 1 dollar to verify it, 10 dollars to clean it up and 100 dollars if it remains incorrect (Fig. 1).
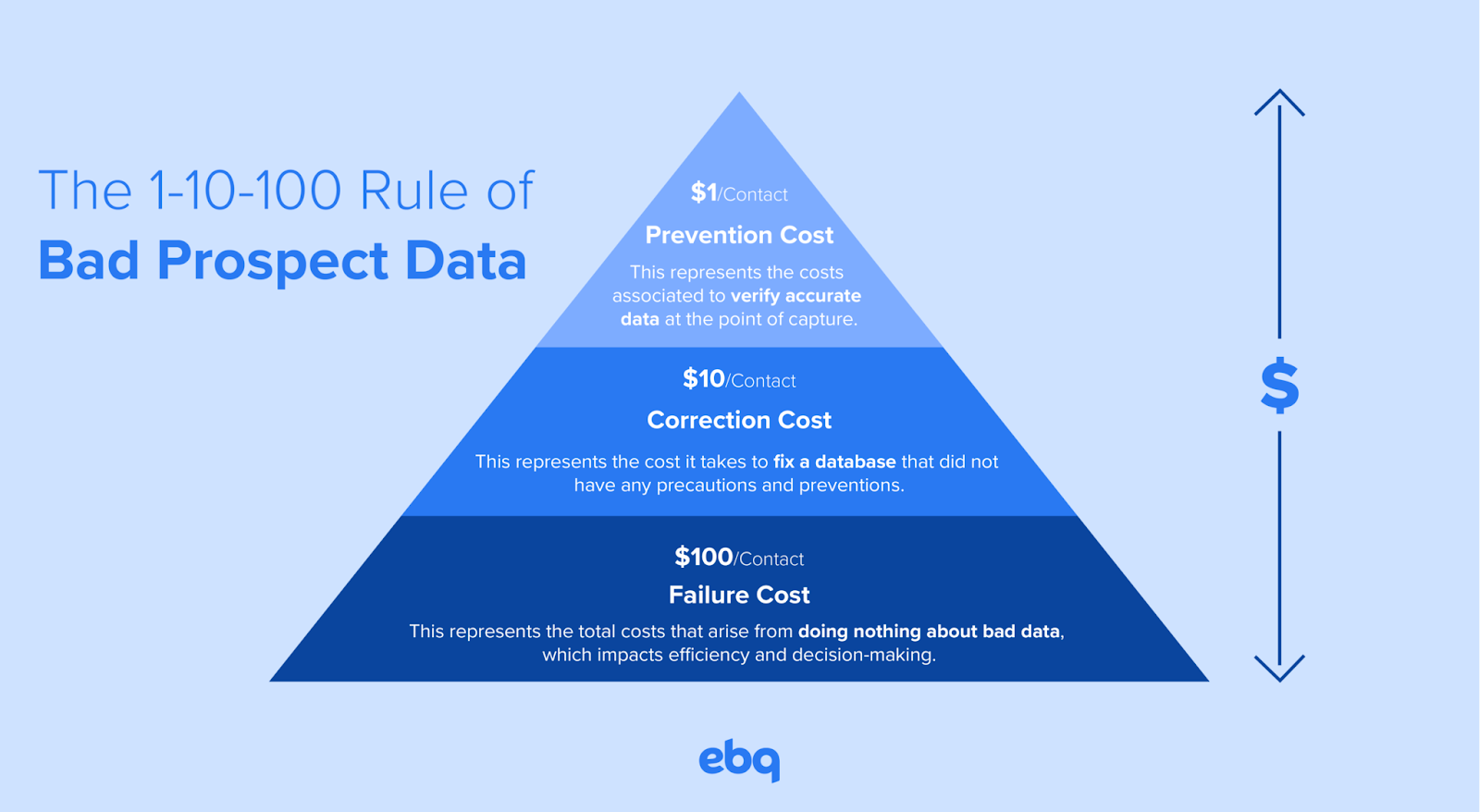
(Figure 1: The 1-10-100 Rule of Bad Prospect Data, ebq)
Furthermore, poor data quality has far more far-reaching consequences than financial losses. These include impacts on employee confidence in decision-making, customer satisfaction and brand image, productivity losses (e.g. due to additional time needed for data preparation), compliance issues, negative consequences for sales and marketing teams, slowed sales cycles and more. Today, every business runs on data - build a secure foundation. And that means proper data quality management.
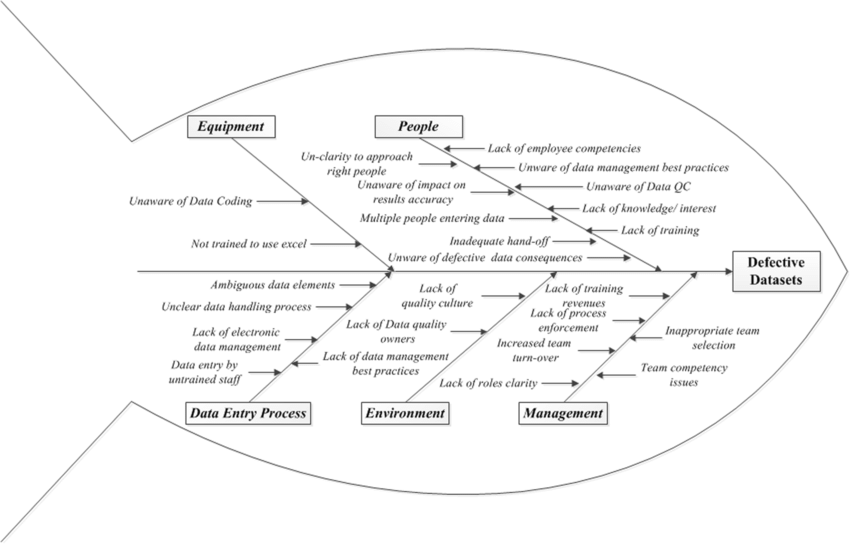
(Figure 2: Root cause analysis of data errors, Researchgate)
What are the causes of poor data quality?
There are many sources of poor data quality, as Fig. 2 illustrates. However, manual human data entry is the most common (see Fig. 3).
1. Manual data entry: When data is entered manually by humans, it is easy to make careless mistakes, typos or inconsistencies. Even small deviations can accumulate in the data and lead to massive quality problems. This is the main reason for low data quality.
2. Outdated data: If data sets are not regularly updated and cleansed, they lose accuracy and relevance over time. Outdated data leads to distorted analyses and incorrect business decisions.
3. Data silos: Isolated data sets in different systems make data consistency and integration extremely difficult. Redundant, contradictory data is the result.
4. Lack of data management: Without clear processes, metrics, roles and responsibilities for data quality management, this critical area remains uncoordinated and neglected. Public bodies or authorities must always appoint a Data Protection Officer (DPO) as part of the GDPR. However, the appointment of a DPO can also be useful for non-obligated companies to ensure appropriate data quality.
5. Complex data sources: The more heterogeneous data sources of different structure and origin that need to be integrated, the more complex data cleansing and harmonisation becomes.
6. System errors: Even small errors or bugs in databases, interfaces or ETL processes can lead to significant data errors on a large scale.
7. Lack of staff qualification: Without training and sensitisation of employees to data quality, this topic remains underestimated and prone to human error. Data must become part of their corporate culture.
Overall, organisations need to take a strategic approach to data quality. This means continuous monitoring, automated rules and an enlightened data culture.
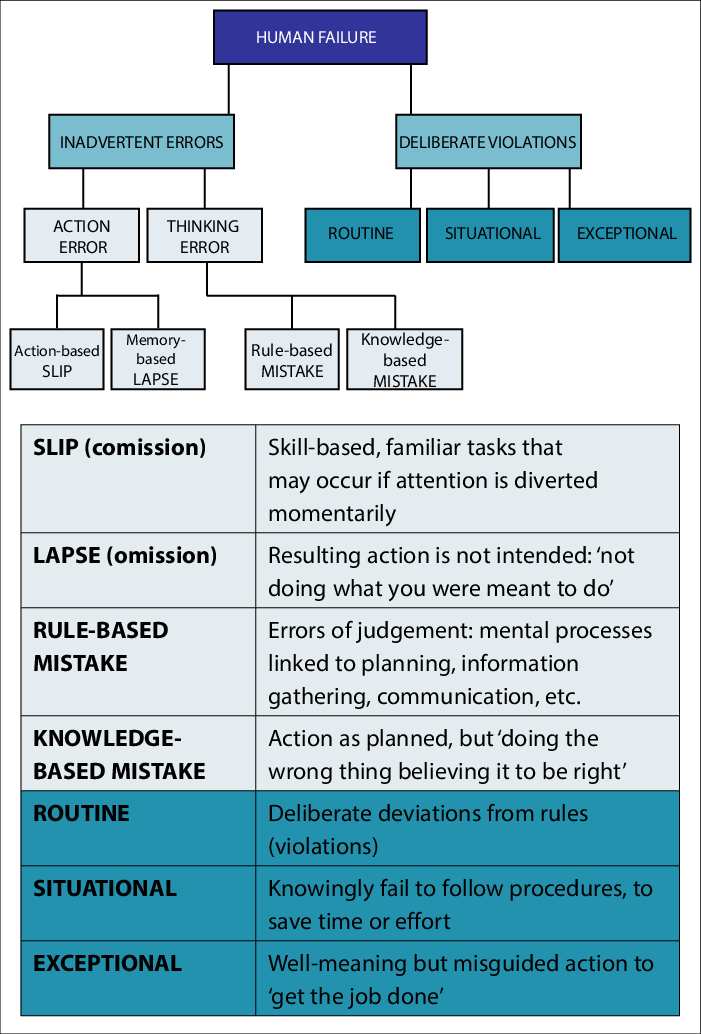
(Figure 3: Human error types, Researchgate)
How is data quality measured?
In practice, there are a large number of criteria that can be used to evaluate the quality of data. The most common evaluation criteria include the following:
- Correctness: Does the data correspond factually with reality?
- Consistency: Does the data from different systems match?
- Completeness: Does the data set contain all the necessary attributes and values?
- Uniformity: Is the data available in the appropriate and same format?
- Freedom from redundancy: Are there no duplicates within the data records?
- Accuracy: Is the data available in sufficient numbers?
- Timeliness: Does the data reflect the current status?
- Comprehensibility: Can each data record be clearly interpreted?
- Reliability: Is the origin of the data traceable?
- Relevance: Does the data fulfil the respective information requirements?
- Availability: Is the data accessible to authorised users?
In general, the criteria of correctness, completeness, consistency, accuracy and freedom from redundancy relate to the content and structure of the data. They cover a large number of the sources of error that are most frequently associated with poor data quality. These mostly include data entry errors, such as typos or duplicate data entries, but also missing or incorrect data values.
What is good enough data quality?
Obviously, the more complete, consistent and error-free your data is, the better. However, it is almost impossible to ensure that all data fulfils the above criteria 100% of the time. In fact, your data doesn't even need to be perfect - instead, it needs to fulfil the requirements or purpose for which the data is to be used.
How good does the quality of the data need to be for a data science project? Unfortunately, there is no universal answer to this question. Depending on the application, there are always individual aspects that affect the required data quality. As already mentioned, these include the purpose for which the data is to be used. This means the use case and the desired modelling method. In principle, the following guidelines should be observed:
1. Purpose-orientated: The data quality must fulfil the specific business requirements and purposes of use. A lower quality may be sufficient for simple operational processes than for critical analyses.
2. Risk assessment: The higher the potential risks and costs of incorrect data, the higher the quality requirements must be.
3. Stakeholder acceptance: Data quality should meet the expectations and minimum requirements of all stakeholders and data users.
4. Balance: A balance must be found between an acceptable level of quality and reasonable effort for data preparation and data cleansing.
5. Continuous improvement: Quality requirements should be regularly reassessed and gradually increased where appropriate.
Ultimately, there is no generalised "sufficient" data quality. This must be defined individually, taking into account utilisation scenarios, costs, risks and the further development of the company. Holistic data quality management is the key.
Which data quality errors can be corrected?
There are different types of data quality errors that need to be treated differently depending on their severity and type. You guessed it: the more difficult the treatment, the more expensive the correction.
- Errors that can be corrected with relatively little effort, e.g. spelling mistakes or duplicate data entries.
- Errors that can be corrected with increased effort, e.g. mixing or deviation of formats.
- Errors that cannot be corrected, e.g. invalid data, missing or outdated entries.
In order to prepare the data successfully, data scientists and the specialist departments must work together to ensure that it is made clear which data is correct and which needs to be corrected. A data dictionary can help to ensure that everyone can understand what is in the data. A data dictionary is an important tool for monitoring and improving data quality. It is a collection of metadata that contains information about the structure, content and use of data.
Even if some errors can be corrected, the better approach is always to prevent them from happening in the first place. Our following best practice checklist will help you to perform an initial quality check on your data.
Data quality management best practice checklist
Here is a short checklist for your data quality management.
Data strategy and data governance
- Define clear data quality goals and metrics
- Appoint data owners and create guidelines
- Establish data protection and security guidelines
Data capture and data integration
- Validate data as it is entered using validation rules
- Eliminate data silos, integrate data sources
- Use master data management (MDM) for consistent master data
Data cleansing and data correction
- Implement deduplication and data synchronisation rules
- Address incorrect, inconsistent and outdated data
- Enrich data with external sources
Continuous control
- Monitor data quality on an ongoing basis
- Carry out regular data audits
- Create data quality reports for management (& stakeholder)
Employees and processes
- Train employees in data quality management
- Implement processes for error reporting and resolution
- Automate data quality routines where possible
Tooling and technology
- Use dedicated data quality tools
- Integrate data quality rules into existing systems
- Utilise data governance and metadata capabilities
Improvement cycle
- Analyse and prioritise data quality issues
- Continuously optimise processes and rules
- Strive for data quality as a core competence
Conclusion
Today, data is considered the fourth production factor alongside land, capital and labour. Data must therefore be regarded as a critical resource that needs to be managed accordingly. To ensure high data quality, a comprehensive data quality management system is mandatory. Data quality is a management task and is by no means the exclusive domain of IT. Data quality is the foundation of the entire data strategy. Various measures are necessary, including initial, one-off measures as well as activities to be carried out on an ongoing basis.
After all, data quality problems not only have an impact on the success of a data science project, but also have far-reaching consequences for the company as a whole. However, the good news for your data science project is that you don't need the perfect data set. And some errors can be corrected by the data scientists during data preparation. However, save yourself the costs and headaches with solid data quality management and our best practice checklist.